Volume 11 - Year 2024 - Pages 397-403
DOI: 10.11159/jffhmt.2024.039
Design, Test and CFD Analysis of Drone for Foreign Object Debris
Haifa El-Sadi1, Massimiliano Orfanini2, Eleonora Orfanini3
3NextEra Energy, Inc. Stuart, FL
2ICO Energy and Engineering, Inc., MA
1Wentworth Institute of Technology 550 Huntington, Boston, MA, USA 02115,
elsadih@wit.edu
Abstract - Runway inspections are regularly conducted by airports to identify and prevent foreign object debris (FOD) that can potentially cause significant damage and pose risks to aircraft. There are an outstanding number of sources that could cause FOD to appear in operational areas, which makes prevention and detection very difficult and time consuming. These sources can come from the environment through wildlife and weather, operating equipment, and personnel on the runways, and even from the airport infrastructure itself. The aim of this project is to assist airports in enhancing runway safety by drones to improve the runway inspection process. SolidWorks, a computer-aided design (CAD) software, was used to create five designs, Given the high stresses that drones must endure during flight, it was decided to use onyx, a composite material made of nylon and carbon fibre, to construct the drone's chassis. Onyx has a strength of up to 40 MPa, The selection of SolidWorks Flow Simulation was employed as the method for conducting the CFD propeller analysis. Subsequent rounds of CFD testing led to the attainment of an average force value of 11.313 N. The outcome closely approximates the manually computed thrust value for the 7035 propellers at an RPM of 13000. The flow simulation findings represent the required thrust magnitude essential for operating the drone at the maximum permissible thrust limit.
Keywords: CFD, FEA, Design, Foreign Object Debris
© Copyright 2024 Authors - This is an Open Access article published under the Creative Commons Attribution License terms. Unrestricted use, distribution, and reproduction in any medium are permitted, provided the original work is properly cited.
Date Received: 2023-12-01
Date Revised: 2024-09-07
Date Accepted: 2024-09-17
Date Published: 2024-11-21
1. Introduction
The Federal Aviation Administration (FAA) stated that Foreign Object Debris (FOD) is any object that is in an improper location in the airport setting that also has the potential to cause damage to equipment and personnel. A background on the topic should first be provided. FOD refers to any unwanted objects present on the runway that can obstruct the path of airplanes, such as screws, bolts, or tire debris that may fall from a landing aircraft. According to the US Department of Transportation's one-year airport study, 60% of the detected FOD on runways consists of metal, while 18% is rubber [1]. According to the U.S. Department of Transportation, the expenses incurred by the airport inspection industry can reach as high as $13.9 billion annually [2]. As stated by the Federal Aviation Administration (FAA), Foreign Object Debris (FOD) is any object that is in an improper location in the airport setting that also has the potential to cause damage to equipment and personnel. There are an outstanding number of sources that could cause FOD to appear in operational areas, which makes prevention and detection very difficult and time consuming. These sources can come from the environment through wildlife and weather, operating equipment, and personnel on the runways, and even from the airport infrastructure itself. The unpredictable origins of FOD have led to a massive number of damaged items in the aerospace industry totalling upwards of $4 billion a year [3]. The aim of this project is to assist airports in enhancing runway safety by drones to improve the runway inspection process. Runway inspections are regularly conducted by airports to identify and prevent foreign object debris (FOD) that can potentially cause significant damage and pose risks to aircraft. FOD can originate from various sources, including weather conditions, fallen or misplaced aircraft components, and the degradation of pavement leading to rubble formation. If these objects remain undetected or are not promptly removed, they can pose serious hazards during take-off or landing. Potential risks include damage to aircraft engines, exterior surfaces, and the surrounding areas affected by debris propelled by the engine during the operation of the planes.
2. Design and CFD Analysis of the Drone
SolidWorks, a computer-aided design (CAD) software, was used to create five designs. The chosen design shown in Figure 1 was remodelled to fit new criteria and goals. These changes can be observed in Figure 2. The propeller guards were removed from the design plan. The final electrical components chosen for the drone required a larger casing, which led to a significant change in the size of the drone's body.
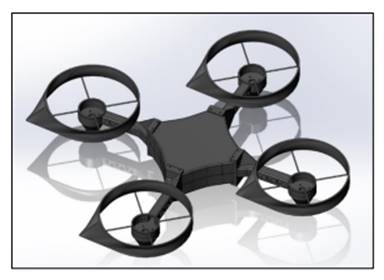
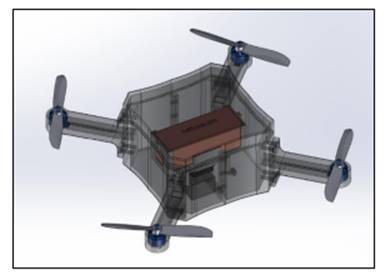
The base plate can be seen in Figure 3, and as shown the components will be mounted below and above the plate resulting in a more time efficient and cost-effective solution that is easy to replace should damage occur.
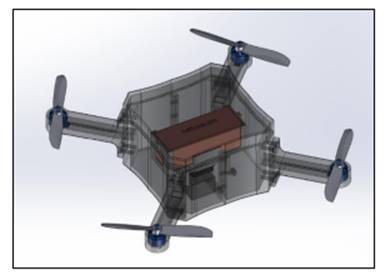
When designing a drone, one of the crucial areas to address is the propellers, which play a pivotal role in the drone's performance. The propellers, which rotate in tandem with the motor, generate the force needed to lift the drone off the ground. To ensure the correct propeller choice and optimal aerodynamic performance, our group employed a combination of hand calculations and CFD analysis, with both results cross-verified for accuracy. Starting with hand calculations, our team relied on fundamental equations that are essential to understanding drone propeller 15 dynamics, which were gathered through the MIT notes catalogue website [5].
The total thrust required to lift the drone off the ground based on its mass.
Where Vp is the propeller velocity, A is the area and 𝜌 is the density. Equation 1 determines the velocity required for the propeller to achieve the target thrust force. Specifically, the equation calculates the vertical distance that the propeller must travel per second. To obtain the desired rotational speed in terms of Rotations per Minute (RPM), the calculated velocity is multiplied by the propeller pitch which measures the vertical distance the propeller covers in one rotation.
2.1 Mathematical Equations and Boundary Conditions
For three-dimensional, incompressible and steady state Newtonian fluid, the continuity equation and the equation of motion are:

Where V is the tangential velocity, and Re is Reynolds number. The CFD package is used to solve Navier-Stokes equations. It enables the use of different discretization schemes and solution algorithms, together with boundary conditions, tangential velocity is 5.364 m/s as shown in Figure 4.
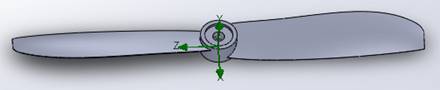
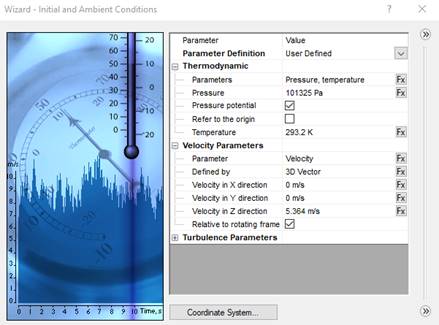
The propeller meshing shown in Figure 5, was executed using a solid mesh with the highest standard refinement level available in SolidWorks, using an element size of 0.035 inches. This process generated a total of 596,144 computational elements. The accuracy of the 20 calculations is paramount, and having a high-quality mesh is crucial to achieving low percentage errors when comparing the simulated and calculated values.
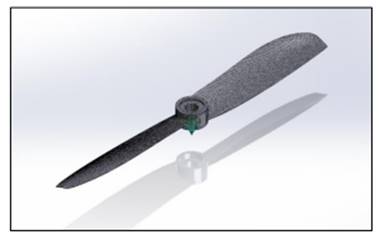
Figure 6 shows the converging graph of propeller’s thrust generation. The simulation successfully computed an accurate force value through a process with convergence to the averaged force value stated to be 11.313 N.
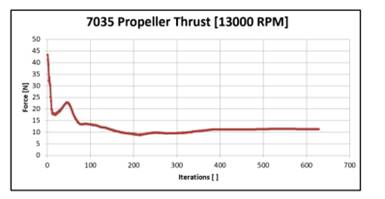
Subsequent rounds of CFD testing led to the attainment of an average force value of 11.313 N. The outcome closely approximates the manually computed thrust value for the 7035 propellers at an RPM of 13000. The flow simulation findings represent the required thrust magnitude essential for operating the drone at the maximum permissible thrust limit. Table 1 lists the results derived from the SolidWorks Simulation.
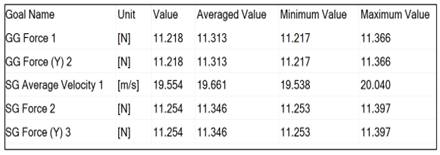
The percentage error was computed to be 0.293%, indicating a high degree of agreement between the theoretical and simulated values. To further evaluate the validity of the Flow Simulation, we conducted simulations at three additional RPM speeds and recorded the results in Table 2. Notably, all four simulations yielded percentage errors of less than 1%, suggesting that our calculations are reliable.
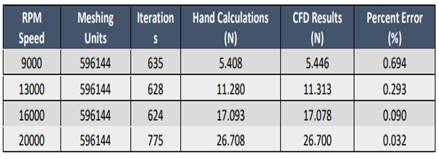
The flow trajectory plot is shown in Figure 7 to illustrate the anticipated airflow patterns caused by the propeller rotation. The highest velocities occur closest to the propeller edge, which is reasonable to infer given the intensity of the air's impact during the propeller's rotation. Additionally, it is noteworthy that the airflow appears to be pushed to rotate counterclockwise, consistent with the direction of propeller rotation specified in the Flow Simulation.
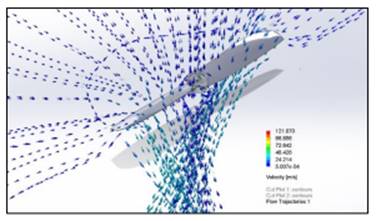
Various plots were generated from the simulation, aiding in our understanding of the interaction between the propeller and airflow. The velocity cut plot shown in Figure 8, depicts the velocity of the air generated by the propeller's rotation. This plot is beneficial in that it allows for the visualization of flow patterns and identification of areas with high or low velocities in the fluid domain. This is crucial in comprehending the design's performance and recognizing any potential issues. The pressure contour plot shown in Figure 9 and 10, represents the pressure distribution along the propeller. Different colours are used to represent the pressure levels at different locations of the model. It is evident that areas of low pressure shown in Figure 9 occur on the top surface of the propeller and the highest pressure shown in Figure 10 at the bottom surface where the air is being dispersed. The highest-pressure point is seen to be at the tips of the bottom face of the propeller, similarly in Figure 7 the highest velocity point is found roughly at the same location, inferring that the velocity and pressure increase with a positive correlation as the propellers have a higher velocity.
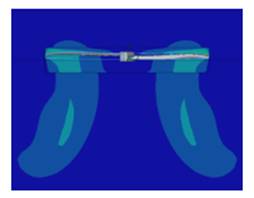
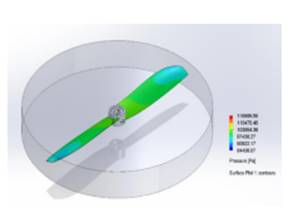
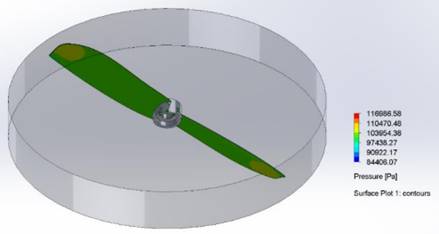
3. Building the prototype
The primary objective is to detect the presence of Foreign Object Debris (FOD) on airport runways. To achieve this, it was crucial to develop a convolutional neural network (CNN) algorithm. The CNN algorithm was trained to recognize a clean runway, which serves as a reference point to identify any foreign objects present on the runway. This approach was suggested by industry expert Barry Bratton, as it is difficult to train the model to recognize every type of FOD that may exist on the runway. The CNN algorithm was trained using a dataset consisting of images of clean runways as well as images containing FOD. CNNs use layers of interconnected nodes to analyse and identify patterns in images. The initial layers of the CNN detect simple patterns, such as edges and curves, while deeper layers detect more complex features. The output of the final layer represents the probability of the input image belonging to each category. During testing, the CNN algorithm processes images captured by the drone's camera and provides a prediction on the presence of FOD on the runway. The accuracy of the algorithm is evaluated by comparing its predictions with ground truth labels assigned to each image. Through this iterative process, the CNN algorithm is optimized to achieve higher accuracy in detecting FOD on airport runways. A dataset of FOD acquired through GitHub [6] and a clear pavement image dataset acquired through Kaggle [7], were used as part of the training. As debris detection is performed by the drone, various images taken in real-time may not match the images the model is trained on. Therefore, when dealing with a small dataset, it is important to augment the data as it makes some modifications to the existing images and allows the model to learn from a wider variety of images. That way, as different scenarios are encountered, the model will be able to make better predictions. The final prototype was assembled, as shown in Figure 11b, however, Figure 11a shows the electronics of the drone
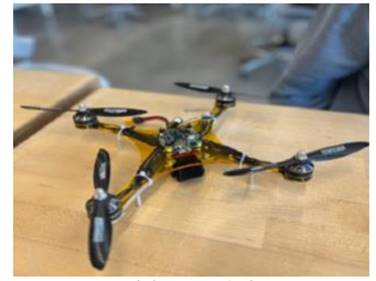
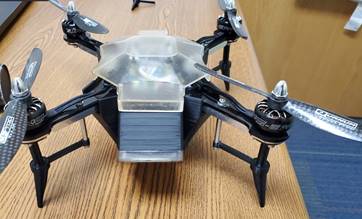
Given the high stresses that drones must endure during flight, it was decided to use onyx, a composite material made of nylon and carbon fibre, to construct the drone's chassis. Onyx has a strength of up to 40 MPa [4] The final design of the drone made of Onyx material with a protective casing surrounding the main board for added durability. Additionally, an extra layer of casing will be incorporated for both aesthetic and aerodynamic benefits. Overall, the final prototype underwent and passed all necessary balancing and flight testing, demonstrating its capability to meet the required manoeuvring standards for successful FOD detection.
4. Flight Test
Three different flight tests were performed to find the optimal power for operation and flight time as shown in Tables 3, 4 and 5. The drone was tested 4 times to get the average flight time.
Table 3: Flight Test at 100% Thrust
Run |
Flight Time |
1. |
14.5 minutes |
2. |
14 minutes |
3. |
13.6 minutes |
4. |
14.2 minutes |
Table 4. Flight Test at 50% Thrust
Run |
Flight Time |
1. |
18.5 minutes |
2. |
18.7 minutes |
3. |
19 minutes |
4. |
18.9 minutes |
Table 5. Flight test at 20% Thrust
Run |
Flight Time |
1. |
21.2 minutes |
2. |
21.6 minutes |
3. |
21.8 minutes |
4. |
22 minutes |
The drone can be operated at 25% power for debris detection. The average time at that power is approximately 22 minutes. This gives the drones deployed plenty of time to survey its assigned segment and take pictures or record live video for debris detection. For the picture method the drone will snap pictures with its camera and send it back to the operator’s computer where the operator will run the picture through a software created using deep machine learning 27 to detect the debris on the runway. Live video method will send a direct video feed to the operator while the drone simultaneously scans for debris using either AI or machine learning.
4. 1. Camera FOD Detection Algorithm
The pictures taken by the drone will be transmitted to the operator via a receiver on their computer. The operators will then run the images sent by the drones through coded software that will be able to tell whether the runway is safe for flight. Additionally, the group will try to code a live image version of this software. This code is just an initial proof that this method exists. With further research and work this technology can give more accurate and faster results. The code was developed using a combination of Python, Tensor Flow libraries, NumPy, and Matplot. Python was used for debugging and running the code. Tensor flow libraries were used to get a pre-trained CNN model. NumPy and Matplot functions were used to process the code. NumPy provided support for numerical operations and Matplot enabled the visualization of data.
There are 2 different data sets. One data set is to verify and detect debris and the other is used to verify a clear runway. This will help the software navigate between whether the runway is clear or needs to be cleaned up.
5. Conclusion
The proposed drone system offers significant advantages over human inspection, as it can scan larger areas at higher speeds and with greater accuracy. This efficiency translates into saved time and energy for personnel currently conducting manual pavement inspections. Furthermore, maintenance of the drone system is simpler, less timeconsuming, and more cost-effective compared to the maintenance of automated FOD detection systems like the X-Sight used in Boston. The estimated maintenance cost for our design is approximately $50 a year per drone. The largest and most impactful benefit of an unmanned aerial vehicle for the use of detection of FOD objects is its ability to ensure runway safety. Whether it is used as an initial detection device or as a secondary verification detection device, a drone utilizing machine learning algorithms can accurately detect FOD in operational areas.
Flow simulation was calculated by simulating three-dimensional Navier-Stokes equations as the method for conducting the CFD propeller analysis of drone. Subsequent rounds of CFD testing led to the attainment of an average force value of 11.313 N. The outcome closely approximates the manually computed thrust value for the 7035 propellers at an RPM of 13000.
The optimal power for operation and flight time has been tested, the drone can be operated at 25% power for debris detection. The average time at that power is approximately 22 minutes.
On the other hand, materials play a critical role in flight testing, therefore onyx, a composite material made of nylon and carbon fibre used to construct the drone's chassis. Onyx has a strength of up to 40 MPa. The final design of the drone made of Onyx material with a protective casing surrounding the main board for added durability.
References
U.S. Department of Transportation. (2009). Airport Foreign Object Debris (FOD) Detection Equipment. Retrieved from
View Article
[2] Transportation Research Board. (2011). Read "Current Airport Inspection Practices Regarding FOD (Foreign Object Debris/Damage)" at NAP.edu. In nap.nationalacademies.org. Airport Cooperative Research Program. Retrieved from
View Article
[3] Bachtel, B. (n.d.). Foreign Object Debris and Damage Prevention. https://www.boeing.com/commercial/aeromagazine/aero_01/textonly/
s01txt.html#:~:text=Foreign%20object%20debris%20(FOD)%20at%20airports
%20includes%20any%20object%20found,industry%20%244%20billion%20a%20 year.
[4] Markforged. “Onyx.” Markforged Support Portal, 17 Oct. 2022,
View Article
[5] Performance of Propellers. 11.7 Performance of Propellers, Accessed 12 May 2023.
View Article
[6] FOD-UNOmaha. (2023, April 24). Foreign Object Debris in Airports (FOD-A) Dataset Overview. Retrieved May 12, 2023, from GitHub website:
View Article
[7] Kaggle. (2023). Pavement Image Datasets. Retrieved May 12, 2023, from www.kaggle.com website:
View Article
U.S. Department of Transportation. (2009). Airport Foreign Object Debris (FOD) Detection Equipment. Retrieved from View Article
[2] Transportation Research Board. (2011). Read "Current Airport Inspection Practices Regarding FOD (Foreign Object Debris/Damage)" at NAP.edu. In nap.nationalacademies.org. Airport Cooperative Research Program. Retrieved from View Article
[3] Bachtel, B. (n.d.). Foreign Object Debris and Damage Prevention. https://www.boeing.com/commercial/aeromagazine/aero_01/textonly/
s01txt.html#:~:text=Foreign%20object%20debris%20(FOD)%20at%20airports
%20includes%20any%20object%20found,industry%20%244%20billion%20a%20 year.
[4] Markforged. “Onyx.” Markforged Support Portal, 17 Oct. 2022, View Article
[5] Performance of Propellers. 11.7 Performance of Propellers, Accessed 12 May 2023. View Article
[6] FOD-UNOmaha. (2023, April 24). Foreign Object Debris in Airports (FOD-A) Dataset Overview. Retrieved May 12, 2023, from GitHub website: View Article
[7] Kaggle. (2023). Pavement Image Datasets. Retrieved May 12, 2023, from www.kaggle.com website: View Article